Table of contents
Introduction to RoseTTAFold
- Developed by: Baker Lab at the University of Washington's Institute for Protein Design.
- Purpose: Predict the 3D structures of proteins from their amino acid sequences.
- It uses generative diffusion-based architectures (one kind of AI model).
- Significance: Enhances understanding of protein functions and interactions, crucial for biological and medical research.
It was first introduced in 2021 and has since undergone significant enhancements.
Key Features
- Machine Learning: Uses deep learning techniques similar to AlphaFold by DeepMind.
- Integration: Combines multiple neural networks to predict protein structures more accurately.
- Accessibility: Open-source model available to the scientific community.
Capabilities
- Three-Track Neural Network: The original RoseTTAFold uses a three-track neural network that integrates one-dimensional, two-dimensional, and three-dimensional data.
- This allows it to predict protein structures with high accuracy and efficiency.
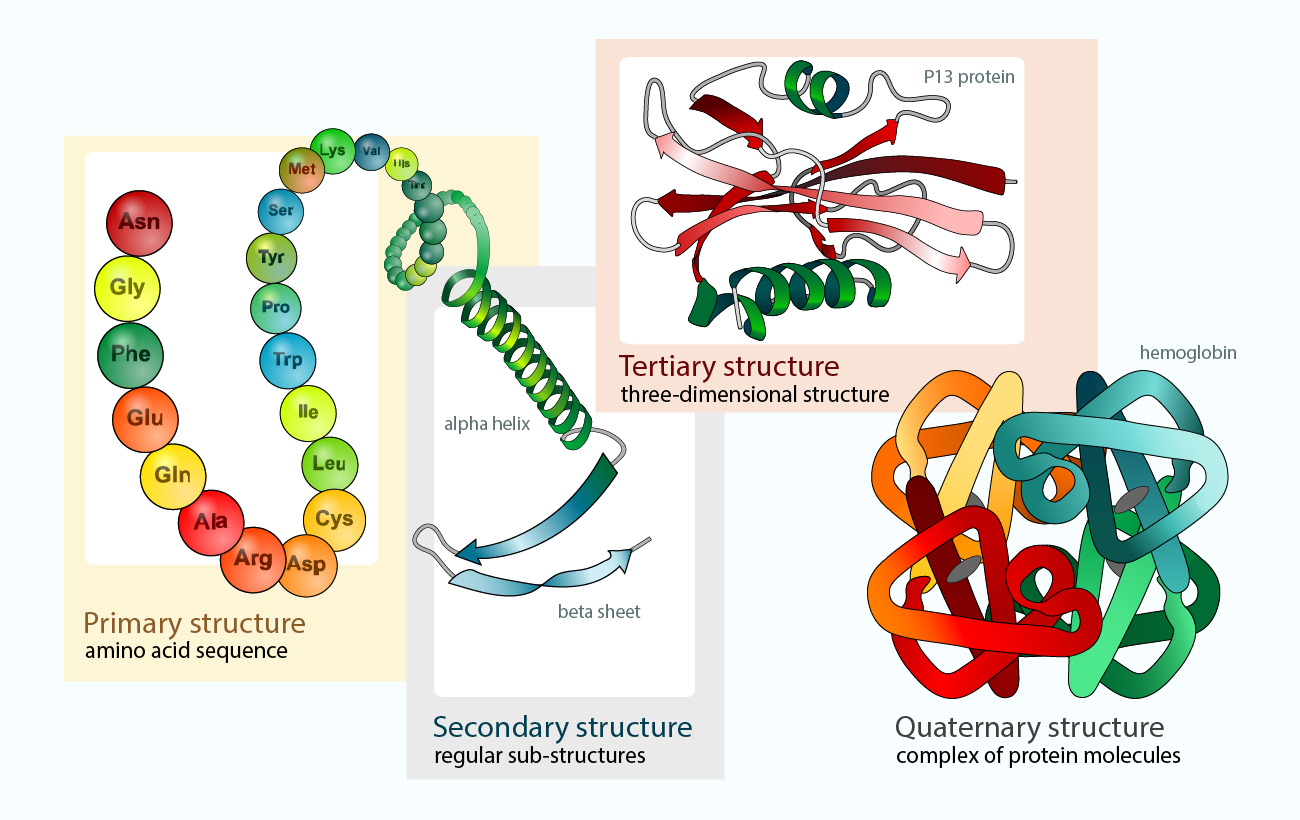
- All-Atom Model: The upgraded version, RoseTTAFold All-Atom, expands its capabilities beyond amino acids to include full biological assemblies.
- This includes proteins, DNA, RNA, small molecules, metals, and other bonded atoms, enhancing the understanding of complex biomolecular interactions.
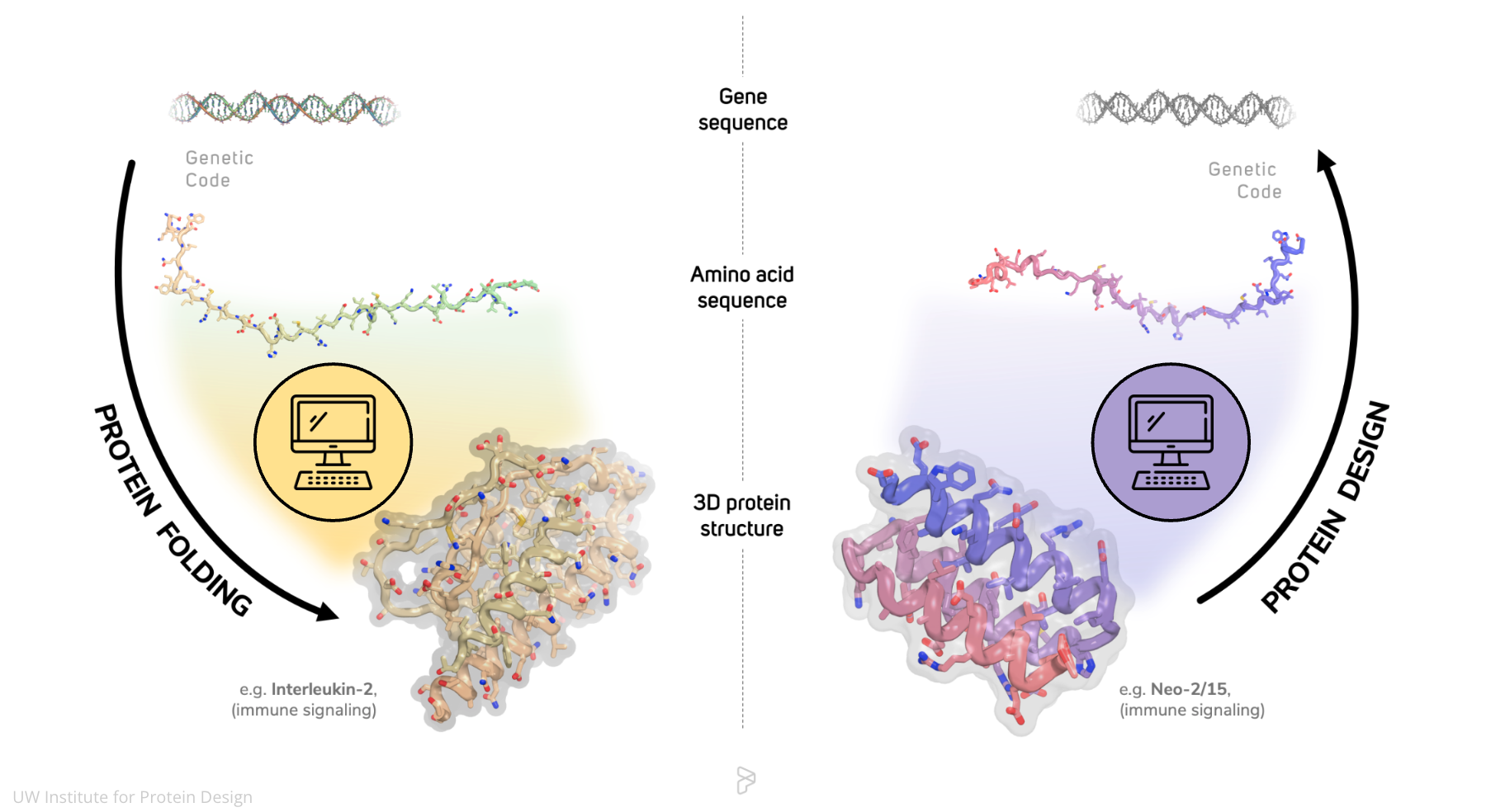

Applications
- Biomedical Research
- Drug Discovery: Helps in identifying potential drug targets by understanding protein structures.
- Disease Understanding: Provides insights into diseases caused by misfolded proteins, such as Alzheimer's and Parkinson's.
- Genomics and Proteomics
- Gene Function Annotation: Assists in determining the function of genes by predicting the structure of their protein products.
- Protein Engineering: Aids in designing proteins with specific functions for industrial and therapeutic purposes.
- Biotechnology
- Enzyme Design: Enhances the development of enzymes for use in industrial processes.
- Agricultural Applications: Helps in designing proteins that can improve crop resilience and yield.
Advantages
- Speed and Accuracy:
- Traditional methods for determining protein structures, such as X-ray crystallography and cryo-electron microscopy, can be time-consuming and resource-intensive.
- RoseTTAFold can predict structures in a fraction of the time, using computational resources that are widely accessible.
- Accessibility:
- Open-Source: The RoseTTAFold code is available on platforms like GitHub, making it accessible to researchers worldwide.
- This democratizes access to advanced protein modeling tools and facilitates widespread use in scientific research.
Future Prospects and Research
- Continued Development: Ongoing enhancements to RoseTTAFold and similar tools aim to improve their accuracy and expand their capabilities.
- Future versions may include more sophisticated models of protein dynamics and interactions with a broader range of biomolecules.
- Collaborative Efforts: The development of RoseTTAFold has been supported by numerous organizations, including the Howard Hughes Medical Institute, Bill and Melinda Gates Foundation, and National Institutes of Health.
- Collaborative efforts and funding are crucial for the continuous improvement and application of these tools in various scientific fields.
Conclusion
RoseTTAFold represents a significant advancement in computational biology, offering rapid and accurate protein structure prediction. Its ability to model complex biomolecular assemblies makes it a powerful tool for researchers in biology and medicine, with the potential to drive significant breakthroughs in understanding and manipulating biological systems.
For UPSC aspirants, understanding the importance and applications of such technologies is crucial for staying abreast of advancements in science and technology.